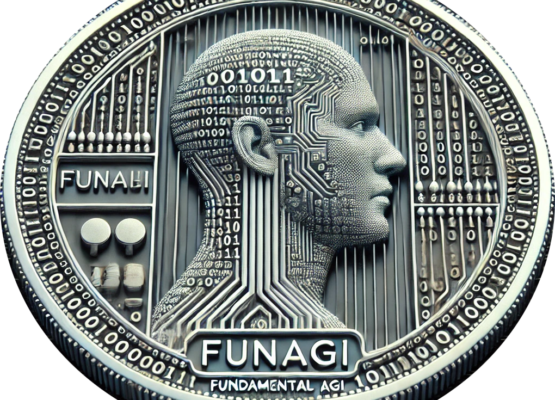
funAGI workflow fundamental autonomous general intelligence framework
The funAGI system is designed as a modular framework for developing an autonomous general intelligence. The workflow integrates several components and libraries to achieve adaptability, dynamic interaction, continuous optimization, and secure data management. Below is a detailed explanation of the funAGI workflow based on the provided files and documentation. 1. Component Initialization 2. Core AGI Logic 3. User Interaction 4. Reasoning and Logic 5. API and Integration 6. Communication and Interaction 7. Installation and Requirements […]
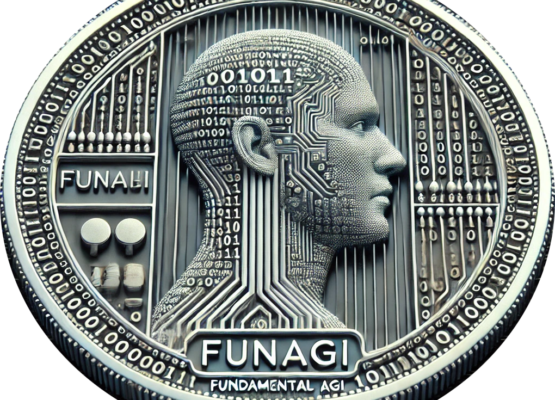
draw_conclusion(self)
ezAGI fundamental Augmented General Intelligence draw_conclusion(self) method The draw_conclusion method is designed to synthesize a logical conclusion from a set of premises, validate this conclusion, and then save the input/response sequence to a short-term memory storage. This function is a critical component in the context of easy Augmented General Intelligence (AGI) system, as it demonstrates the ability to process information, generate responses, validate outputs, and maintain a record of interactions for future reference and learning. […]

Understanding SocraticReasoning.py
understandin the ezAGI framework requires a fundamental comprehension of reasoning with SocraticReasoning.py disclaimer: ezAGI fundamental Augmented Generative Intelligence may or not be be fun. use at own risk. breaking changes version 1 To fully audit the behavior of how the premise field is populated in the SocraticReasoning class, we will: SocraticReasoning.py Audit Initialization and setup of SocraticReasoning class Adding Premises Programmatically Adding Premises Interactively Now, let’s look at the interactive part of the interact method: […]